🚀 What's Lead & account scoring:#
Lead & account scoring ranks those entities in order based on their likelihood of becoming customers. It can be composed of several data: firmographic, momentum-based (intents), persona-based, behavioral, and technographic to grade the contact or account object in the most exhaustive way possible and enable sales and marketing to spend resources more efficiently.
Have you ever wondered why lead and account scoring is crucial for B2B companies?
Well, it's simple - It helps sales and marketing teams identify and prioritize the best prospects.
In today's fast-paced world, where reaching out to audiences across multiple channels and mediums has become a norm, the ability to surface high-priority leads is essential to ensure that we allocate our resources where it matters the most.
According to the wise words of Chet Holmes, only 3% of any market is actively looking for solutions, while 40% may entertain the idea of switching.
Think about it.
If 97% is not actively in buying mode, having a reliable way to evaluate where you should dedicate your time and sales effort is your competitive advantage.
Flagging a lead as an "MQL" based on a single action, such as subscribing to an online event, is not enough. Instead, a multi-dimensional scoring model is necessary.
It should consider various attributes like technographic, firmographic, behavioral, and psychographic data to assess the conversion probability accurately.
When it comes to modern SaaS companies, we often see a split in the scoring model between a "fit score" and a "behavioral or activity score.”
- The fit score assesses how well the lead matches your ideal customer profile based on company and person attributes

- The behavioral or activity score measures the prospect's engagement with
your company and decays over time to ensure that you only consider recent and
active behavior.

‍It makes more sense to split between intrinsic and intent attributes. This provides greater granularity to drive unique sales and marketing actions.
Most of the time, you have a grade (A, B, C) combining the two scoring into one, and different SLAs to engage with each of them like A= in the 5 minutes, B= in the next two hours, C= in the day. This is often made through lead routing.‍
At Revenue.io, they do things differently by using a combined score that informs how you should engage the lead: 1:M (one-to-many) for low-value accounts, 1:F (one-to-few) for average accounts, and 1:1, a one-to-one approach for high-value accounts.
One thing to keep in mind is that you need to ensure that you have enough capacity for each cohort. You don't want to overwhelm your reps with too many high-value accounts and not enough time to give them the attention they deserve.
This refers to the famous 3VC for “Volume, Value, Velocity, and Conversion”. These metrics give us a glimpse into the sales pipeline performance & revenue generation. Make sure to compare these metrics to previous months, quarters, or years for each cohort to understand how you got to that revenue.‍
OK, now let’s get started.
Data over intuitions‍#
So where do you start?
The first step is to analyze your current and potential customers and identify common patterns.‍
This requires a clear understanding of your Ideal Customer Profile and the success criteria that lead to a conversion.
Building a scoring model involves collaboration from all stakeholders, especially the sales team, who are customer-facing and can provide valuable insights into deciding which factors to use, how to weigh them, and knowing the blockers in the purchase decision.‍
When it comes to identifying potential leads, it's not just about their firmographic and person-based attributes.
You may want to consider technographic factors, as your solution integrates well with your prospect's existing stack. It could be around outreach criteria, like noticing that having multiple stakeholders in the loop maximizes the conversion probability. (it’s often the case, that’s what we call triangulation)

The same goes If you noticed that leads who requested a demo are 2x times more likely to close than leads who don't or that you have a greater win rate on accounts belonging to a specific industry, then you could award points to all accounts with those attributes.
You get the idea.‍
You know what's worse than having no scoring model at all?
Having a bad one!
So don’t rush it. It's crucial to take your time and approach the process carefully.
Start by identifying 10 to 20 data points you know work, and use them to build a reliable minimum viable product (MVP). Don't try to create a highly sophisticated scoring model right off the bat - that could lead you down a path of confusion and uncertainty.
A good place to start is with a simple spreadsheet to get everyone aligned on the attributes to use. This way, you can be confident that you have a solid foundation to build upon. Below is a simple template to let you visualize the logic. Of course, it is just an example, you should tailor the scoring and adapt it according to your business specific needs.
đź’ˇ Spreadsheet template
If you've identified the components that matter most for your scoring model, Congratulations!
But don't stop there.‍
The next step is to estimate each attribute's relative impact on the lead's value and weigh them accordingly.
For example, for the technographic:
- Simple: +1 point for marketing automation software
- Weighted: +3 points for Marketo because we have a direct integration vs. +1 for Salesforce marketing cloud because there is no integration yet.
Now, the final step is to settle the sales readiness - the point at which an account is considered ready to be assigned to a salesperson. This threshold could be set at 100, for example.
This will be particularly helpful in managing the handover from marketing to sales and foster greater alignment.
But remember, this is an ongoing process - you'll need to make tweaks and adjustments as your business evolves and your goals change. Stay flexible and continue to refine your scoring system for more accurate measurements over time.
💡 Tips: Once you run your first scoring model, let’s say after one month, I strongly recommend asking the data team to do a report on the scoring attached to an account and see the related closing rate achieved to make sure there is evidence in correlating the score with a higher closing rate.‍
Manual vs. predictive scoring models‍#
Now that you have your criteria in place and have determined the relative weight for each attribute, it's time to consider the different scoring models available.‍
It's important to keep in mind that not all scoring systems are created equal.
The first type is the manual scoring model, where you manually award or deduct points for specific attributes based on a configured list of positive and negative factors.
For instance, if you're a spend management solution and your target company is accompanied by an external accounting firm, you may want to mark it as a negative attribute, as they can prevent the sales from moving forward. The same logic applies if the prospect is already using a solid competitor.
Another common use case is that you don’t serve specific countries, so you want to subtract points from contacts based on those areas.

The second type is predictive scoring, which uses regression analysis as the most common model creation method.
Instead of having a static score, predictive scoring use AI to review your historical data, calculate similarities across your customers and cross-reference that information against leads that failed to close. It removes the possibility of human error or bias and relies on hard data to make predictions - but it can also be a black box.
While on the paper, I agree it looks much sexier than manual scoring, the main limit for B2B SaaS is having enough data. The datasets are often too small to warrant anything reliable.‍
Types of Lead Scores‍#
While most of the scoring models in the B2B space are lead-account based, you can have scoring models for different use cases beyond the one used to assess the closing/converting probability.
The most common would be:
Expansion scoring:

‍The idea is to focus on generating more revenue from your existing customers by identifying cross-sell or upsell opportunities. The scoring takes into consideration things like the number of users/seats, the use of your product, and even third-party data like employee growth or new hires for instance.
Churn prediction:

It's often said that customer retention is more important than acquisition. The idea is to proactively identify signals of disengagement from your customers and score them to prevent churn. These signals can include decreased usage or engagement, more delay in paying each month, or increased negative feedback.
Product Adoption:

Here, we want to draw the path to the “Aha moment” and make sure your customers use the most relevant features for their use cases. The key is to identify the characteristics that make your solution successful in the eyes of your most important customers. You can have similar scoring to track feature adoption or onboarding effectiveness.
Alright, guys!‍
Now, you might be wondering, what’s the best place to implement those scoring models?‍
Scoring Models Implementation: CRM or Data Warehouse?#
Scoring model in your CRM or MAP‍
Lead scoring is foundational as an efficiency driver but often falls short due to data challenges. It's not uncommon to have important customer data that doesn’t natively live within the platform, making it harder to build an accurate scoring model.
Indeed, the next most important pitfall when implementing lead scoring, beyond not having a clear methodology (what we talked about during the 1st part), is not having exhaustive & reliable data.

As they say in the data world, "garbage in, garbage out."
Yes! The quality of the inputs will always determine the quality of the output.
That’s where CRM & Marketing Automation Platforms can present some limits.
The main ones in the majority would be around CRM/MAP hygiene:‍
- Duplicated records: We often find CRMs containing duplicate records with conflicting information, and we struggle to know which one to prioritize in an automated way.
- Inaccurate/ Outdated information: The first reason is that you usually have in your CRM information that dates from when you create the account record but is rarely updated. Â The most common is upsell that happens, and we still have the first MRR when we convert the company.
- Incomplete data: Your sales team must manually input a big part of the data, resulting in a lack of consistency & missing fields. Examples include incomplete sales notes, missing deal next steps, and unqualified reasons.
- Siloed: Â It can be tough to capture behavioral data and key events on your website or app, which can be crucial in understanding your customer's needs and behavior. This information is often unavailable in your CRM, making it challenging to get a complete picture of your customer's journey.
One of the most significant hurdles is accessing all the data in your CRM. This often requires manual CSV downloads and uploads or complex data pipelines that connect multiple tools and map the data to custom properties in your CRM. While it may sound simple, executing correctly can be incredibly challenging.‍
In my experience, custom objects quickly become a mess.
Why?
Because you are fighting with rigid and proprietary architecture.
Instead, data warehouses offer flexibility, scalability, and security, making them an excellent solution for managing large amounts of data. Plus, it's likely that all your data is already stored in your data warehouse‍
360 scoring in your data warehouse#
When you build a scoring model within your data warehouse, you have access to the full range of your customer data, which can be incredibly valuable.‍
Your data warehouse likely contains data from multiple sources, including your CRM, marketing automation platform, customer success tools, behavioral tracking, product analytics, and more.‍
The only actionable 360-degree view of your customer lives in your data warehouse.
The main benefits of doing scoring models on top of the data warehouse are:‍
- Greater flexibility: A data warehouse provides more flexibility in data storage and manipulation compared to a CRM system. You can store and access data from multiple sources, perform complex calculations and transformations on the data, and create custom entities (ie, workspaces) that fit your business needs.
- Improved accuracy: Lead and account scoring algorithms rely on a variety of data points and factors to make predictions. By leveraging all the data from your warehouse, you would be able to improve the accuracy of your scoring models compared to relying solely on data available from your CRM.
- Scalability: Data warehouses are designed to handle large amounts of data and can easily scale to accommodate growing datasets.
- Faster processing: Data warehouses are optimized for fast processing of complex queries and aggregations. This can be especially useful when dealing with large datasets and complex scoring models.
- Integration with BI tools: Data warehouses can be integrated with a wide range of data visualization tools, making it easier to create custom reports to track the correlations between the scoring & the conversion rate for instance.
As a consequence, data warehouses are today the single source of trust for data-driven companies, used for two main use cases - building reports to drive business insights and orchestrating revenue operations.
This is where Cargo comes in. We enable business folks to take advantage of the wealth of data from the warehouse, to build scoring models based on exhaustive and reliable data. You can further enrich data using third-party providers like Clearbit or Cognism within the platform and seamlessly sync the data back to your CRM or marketing automation platform.

We also provide the ability to write back to your data warehouse, enabling your data teams to access custom fields created within Cargo and push them back to the data warehouse (on a separate table, though).

👉 Interested in building scoring models on top of your data warehouse? Signup here, and we'll get you onboard.
‍
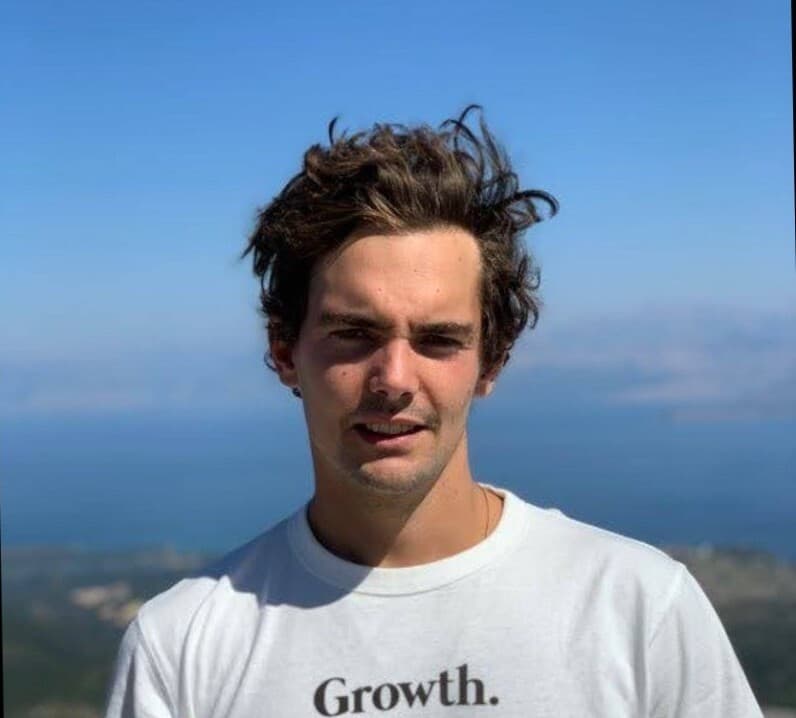